Applied Regression Analysis: A Masterful Guide to Regression Techniques in Probability and Statistics

Applied regression analysis is a powerful statistical tool that allows researchers to investigate the relationships between variables and predict outcomes. It is widely used in various fields, including business, economics, healthcare, and social sciences. This comprehensive guide delves into the intricacies of Applied Regression Analysis, providing a thorough explanation of the fundamental concepts, methodologies, and applications of this essential statistical technique.
Regression analysis is a statistical method that aims to establish a relationship between a dependent variable and one or more independent variables. The goal is to develop a model that can accurately predict the value of the dependent variable based on the values of the independent variables. Regression models are widely used for forecasting, decision-making, and understanding the underlying mechanisms that govern complex phenomena.
Applied regression analysis extends the fundamental concepts of regression analysis by equipping researchers with the tools and techniques necessary to tackle real-world problems. This involves understanding the different types of regression models, selecting appropriate variables, interpreting results, and dealing with common challenges encountered in regression analysis.
4.1 out of 5
Language | : | English |
File size | : | 38533 KB |
Text-to-Speech | : | Enabled |
Screen Reader | : | Supported |
Enhanced typesetting | : | Enabled |
Print length | : | 737 pages |
Applied regression analysis covers a diverse range of regression models, each tailored to specific research objectives. Some commonly used models include:
- Linear Regression: A fundamental model that establishes a linear relationship between a dependent variable and independent variables.
- Nonlinear Regression: Extends linear regression by allowing for curvilinear relationships between variables.
- Logistic Regression: Employs a logistic function to model the probability of a binary outcome.
- Poisson Regression: Used to model count data that follows a Poisson distribution.
- Multivariate Regression: Incorporates multiple independent variables to predict the dependent variable.
Selecting the right variables is crucial for developing effective regression models. Applied regression analysis provides guidelines for variable selection, including:
- Theoretical Considerations: Selecting variables that are logically related to the dependent variable based on theory and prior knowledge.
- Statistical Significance: Using statistical tests to identify variables that significantly contribute to the predictive power of the model.
- Collinearity Assessment: Avoiding highly correlated variables that can lead to inflated standard errors and unstable parameter estimates.
The interpretation of regression analysis results is a key step in understanding the implications of the model. Applied regression analysis focuses on:
- Coefficient Estimation: Determining the magnitude and direction of the relationship between independent and dependent variables.
- Hypothesis Testing: Evaluating the statistical significance of the estimated coefficients to assess their reliability.
- Confidence Intervals: Constructing intervals around the estimated coefficients to provide a range of plausible values.
- Model Evaluation: Assessing the goodness-of-fit of the regression model using various metrics such as R-squared and adjusted R-squared.
Applied regression analysis also addresses common challenges encountered during model development:
- Outliers: Identifying and dealing with data points that significantly deviate from the expected pattern.
- Multicollinearity: Handling the presence of highly correlated independent variables that can cause estimation problems.
- Heteroscedasticity: Addressing the issue of non-constant variance in the residuals.
- Autocorrelation: Detecting and correcting for the presence of serial correlation in the residuals.
Applied regression analysis has far-reaching applications across diverse fields:
- Business: Demand forecasting, market segmentation, and customer behavior analysis.
- Economics: Economic growth modeling, inflation prediction, and labor market analysis.
- Healthcare: Disease risk assessment, treatment efficacy evaluation, and patient outcome prediction.
- Social Sciences: Social mobility modeling, educational attainment analysis, and public policy evaluation.
Applied regression analysis is an indispensable tool for researchers seeking to uncover the relationships between variables, predict outcomes, and gain insights into complex phenomena. Its comprehensive framework provides a robust foundation for making informed decisions, formulating effective policies, and advancing knowledge across a multitude of disciplines.
This exploration of Applied Regression Analysis has illuminated its fundamental principles, methodologies, and applications. By mastering the techniques outlined in this comprehensive guide, researchers can harness the power of regression analysis to address complex research questions, advance their understanding of the world, and make a meaningful impact on their respective fields.
4.1 out of 5
Language | : | English |
File size | : | 38533 KB |
Text-to-Speech | : | Enabled |
Screen Reader | : | Supported |
Enhanced typesetting | : | Enabled |
Print length | : | 737 pages |
Do you want to contribute by writing guest posts on this blog?
Please contact us and send us a resume of previous articles that you have written.
Text
Story
Reader
Library
E-book
Magazine
Sentence
Shelf
Bibliography
Preface
Synopsis
Footnote
Manuscript
Codex
Tome
Bestseller
Narrative
Biography
Reference
Encyclopedia
Narrator
Character
Resolution
Catalog
Stacks
Archives
Periodicals
Study
Reserve
Reading Room
Rare Books
Interlibrary
Study Group
Thesis
Dissertation
Awards
Reading List
Book Club
Theory
Textbooks
Susan Gillis
Tracey Bryant
Jason Blair
Carmela Carr
Marisa Chappell
David R Mayhew
Paulette Kouffman Sherman
J Nathan
Jeff L Creasy
Carlos R Abril
Dick King Smith
Julie Knutson
August Farrow
Don Jessop
Sue Rusch
Deepak Singh
Sally Lloyd Jones
Allan R Wallace
Alice Kinerk
Noemi Lois
Light bulbAdvertise smarter! Our strategic ad space ensures maximum exposure. Reserve your spot today!
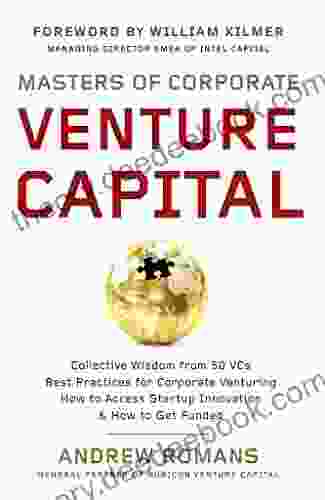

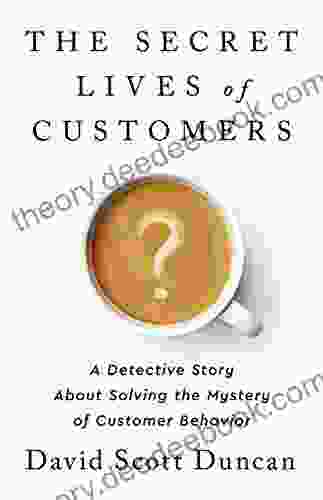

- Phil FosterFollow ·14.5k
- Fernando PessoaFollow ·14.5k
- Jacob HayesFollow ·11.2k
- Morris CarterFollow ·6.7k
- Colin RichardsonFollow ·4.3k
- Glenn HayesFollow ·9k
- Alexander BlairFollow ·10k
- Enrique BlairFollow ·15k
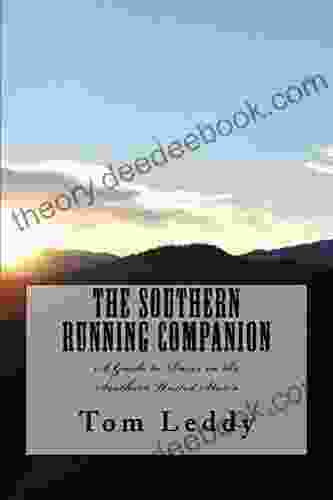

An Extensive Guide to Road Races in the Southern United...
Welcome to the...
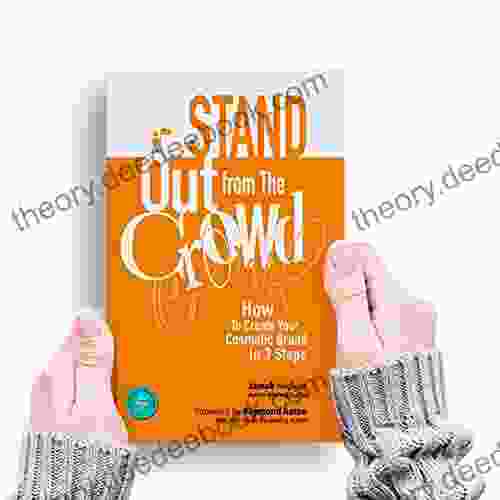

How to Create Your Cosmetic Brand in 7 Steps: A...
The cosmetic industry is booming, with an...
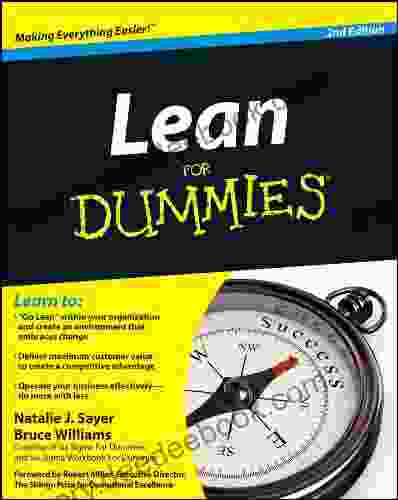

Lean for Dummies: A Comprehensive Guide to the Lean...
Lean is a management...
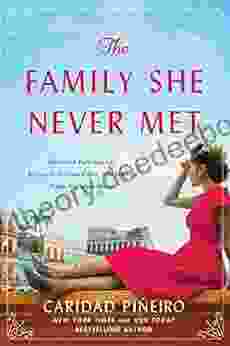

The Family She Never Met: An Enthralling Novel of...
Prologue: A Serendipitous...
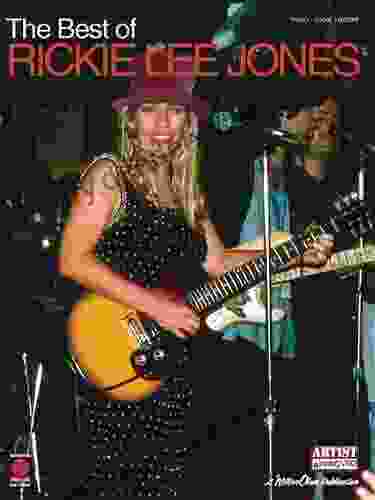

The Alluring Soundscape of Rickie Lee Jones: A Journey...
: The Enigmatic Soul of...
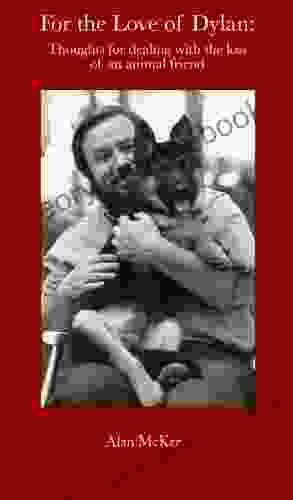

For The Love Of Dylan: An Exploration of Bob Dylan's...
Bob Dylan, the...
4.1 out of 5
Language | : | English |
File size | : | 38533 KB |
Text-to-Speech | : | Enabled |
Screen Reader | : | Supported |
Enhanced typesetting | : | Enabled |
Print length | : | 737 pages |